Seeking to minimise the challenge that recidivism represents to justice systems, a new bias-free AI system for predicting reoffending is being developed by an IPS partnered initiative. Aligned with EU legislation for non-discriminatory AI, this new system will help identifying which rehabilitation interventions should be prioritised to reduce the risk of recidivism more effectively.
Recidivism remains a complex issue, with definitions varying across jurisdictions and influenced by factors like substance abuse, mental health, and social ties. Measured through rearrest, reconviction, or reincarceration, it shapes criminal justice policies. Effective rehabilitation programmes addressing dynamic risk factors are key to breaking the cycle of reoffending.
Recidivism refers to the tendency of individuals to re-engage in criminal behaviour after having been punished or rehabilitated for a prior offence. While this concept is widely discussed, its exact definition varies significantly across jurisdictions and contexts. At the European level, efforts have been made to enhance semantic clarity, but the complexity of recidivism has prevented the establishment of a universally accepted definition. Recidivism can manifest in three main ways:
i. Rearrest: Refers to situations in which the police authorities arrest an individual for a new offence. While this measure is straightforward for data collection, it does not consider the presumption of innocence until proven guilty, which makes verification of the commission of a crime dependent on conviction by a court ¹.
ii. Reconviction: Refers to the formal process of being found guilty of a new crime in a court of law. This measure provides a more definitive indication of criminal behaviour; However, it introduces a high degree of complexity for data collection purposes ².
iii. Re-incarceration: Involves the return of an individual to prison or jail due to a new conviction or a breach of parole or probation conditions. This is particularly useful for assessing rehabilitation efforts but does not account for criminal cases that do not result in prison sentences ³.
The choice of definition significantly influences how recidivism is measured and understood, shaping policies and interventions aimed at reducing repeat offences.
Factors Influencing Recidivism
Addressing recidivism requires a thorough understanding of the factors contributing to repeated criminal behaviour. Researchers classify these factors into two categories ⁴:
- Static risk factors: Unchangeable characteristics such as age, gender, and prior criminal history. These are useful predictors but cannot be modified through intervention.
- Dynamic risk factors: Modifiable factors such as substance abuse, unemployment, or mental health issues. These can be addressed through targeted rehabilitation programmes to reduce recidivism rates.
The Role of Rehabilitation in Reducing Recidivism
Successful rehabilitation programmes target dynamic risk factors, helping individuals reintegrate into society and reducing their likelihood of reoffending. Education, vocational training, mental health support, and substance abuse treatment have all been shown to lower recidivism rates ⁸.
The FAIR-PReSONS Project: Advancing AI for Fair Recidivism Prediction
Considering the increasing use of artificial intelligence (AI) in criminal justice decision-making,the FAIR-PReSONS project aims to develop a bias-free AI system for predicting recidivism. Aligned with EU legislation for non-discriminatory AI, this initiative recognises the risks posed by biases inherent in data and algorithms. Variables such as gender, race, and nationality can significantly influence AI-driven decisions, raising concerns about fairness and equity.
As part of this project, a comprehensive range of national data is being collected, encompassing diverse variables. This will help us understand the relationship between the different variables with the risk of reoffending and the identification of the most influential factors in each context. Ultimately, this process is a stepping stone towards identifying which rehabilitation interventions should be prioritised to reduce the risk of reoffending more effectively.
By combining data collection from prisons and criminal justice organisations with an emphasis on fairness, the FAIR-PReSONS project aims to contribute to more just and effective criminal justice policies.
References
¹ Boxer, P., Docherty, M., Ostermann, M., Kubik, J., & Veysey, B. (2017). Effectiveness of multisystemic therapy for gang-involved youth offenders: One year follow-up analysis of recidivism outcomes. Children and Youth Services, 73, 107–112
² Baglivio, M. T., Wolf, K. T., Piquero, A. R., DeLisi, M., & Vaughn, M. G. (2017). Examining parental drug and mental health problems, and markers of neuropsychological deficits among serious juvenile offenders. Criminal Justice and Behavior, 44(8), 1009–1029
³ Benda, B. B., & Toombs, N. J. (2002). Two preeminent theoretical models: A proportional hazard rate analysis of recidivism. Journal of Criminal Justice, 30, 217–228
⁴ Yukhnenko, D., Blackwood, N., & Fazel, S. (2020). Risk factors for recidivism in individuals receiving community sentences: a systematic review and meta-analysis. CNS spectrums, 25(2), 252–263.
⁵ Bonta, J., Law, M., & Hanson, K. (1998). The prediction of criminal and violent recidivism among mentally disordered offenders: A meta-analysis. Psychological Bulletin,123,123–142.
⁶ Bronson, Stroop, Zimmer, & Berzofsky. (2017). Drug Use, Dependence, and Abuse Among State Prisoners and Jail Inmates, 2007-2009. U.S. Department of Justice Office of Justice Programs Bureau of Justice Statistics.
⁷ Blanchette, K. (2002). Classifying female offenders for effective intervention: Application of the case based principles of risk and need. Forum on Correctional Research,14, 31–35
⁸ Kimmitt, S. (2011). The impact of community context on the risk of recidivism among parolees at one , two-, and three-year follow-ups. Ohio State University.
Learn more about this project
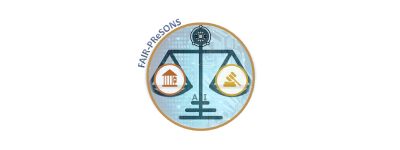
FAIR PRESONS
Fair predictions of gender-sensitive recidivism
To learn more about this initiative, visit its project page.
Related projects
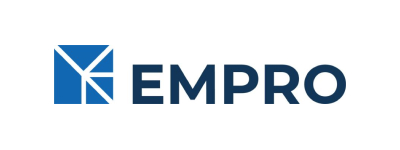
EMPRO
Empowerment of the Framework Decisions 2008/947 and 2009/829 through EU Probation Services
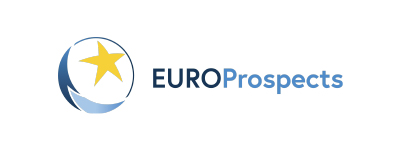
EUROProspects
Ensuring Probationers’ Rehabilitation Prospects via targeted enhancement of the implementation of FD 2008/947
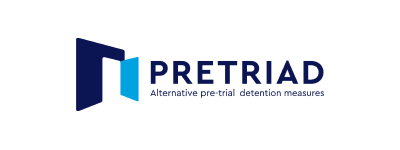
PRE-TRIAD
Alternative pre-trial detention measures: Judicial awareness and cooperation towards the realisation of common standards
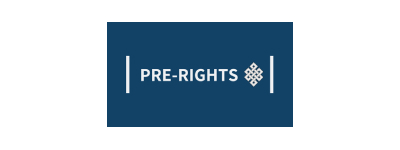
PRE-RIGHTS
Assessing the impact and performance of preventive measures on EU Directives and Framework Decisions
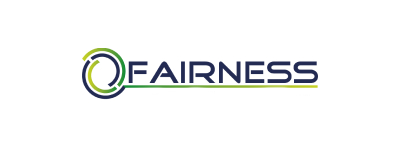
FAIRNESS
Implementation of the Stockholm’s Roadmap in cases of terrorism and radicalisation
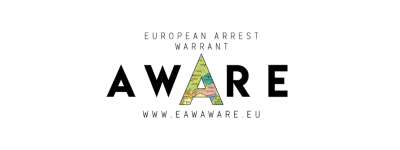
AWARE EAW
European Arrest Warrant
Related news
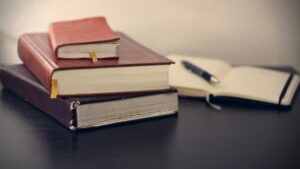
Creating a more effective and harmonised application of the Framework Decision 2008/947 to improve rehabilitation prospects
Read More »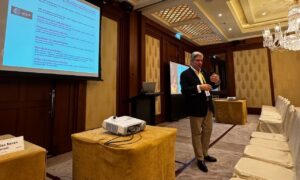
HORUS 360 iOMS illustrates how data-driven intelligence enhances safety and efficiency in corrections at Smart Prisons event
Read More »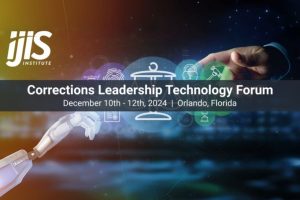
ICJS and IPS Showcase HORUS 360 iOMS at the 2024 IJIS Institute Corrections Technology Leadership Forum
Read More »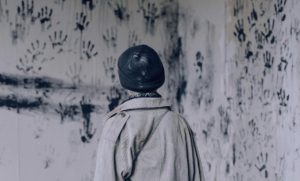